Intelligent Design of Autonomous Materials
The Intelligent Design of Autonomous Materials Group welcomes competitive and enthusiastic applicants to conduct cutting-edge research at HKUST. Interested persons with theoretical or computational background in Applied Mathematics, Physics, Biophysics, Materials Science, Mechanical Engineering, or Chemical Engineering are encouraged to send enquiries to Rui's email address at
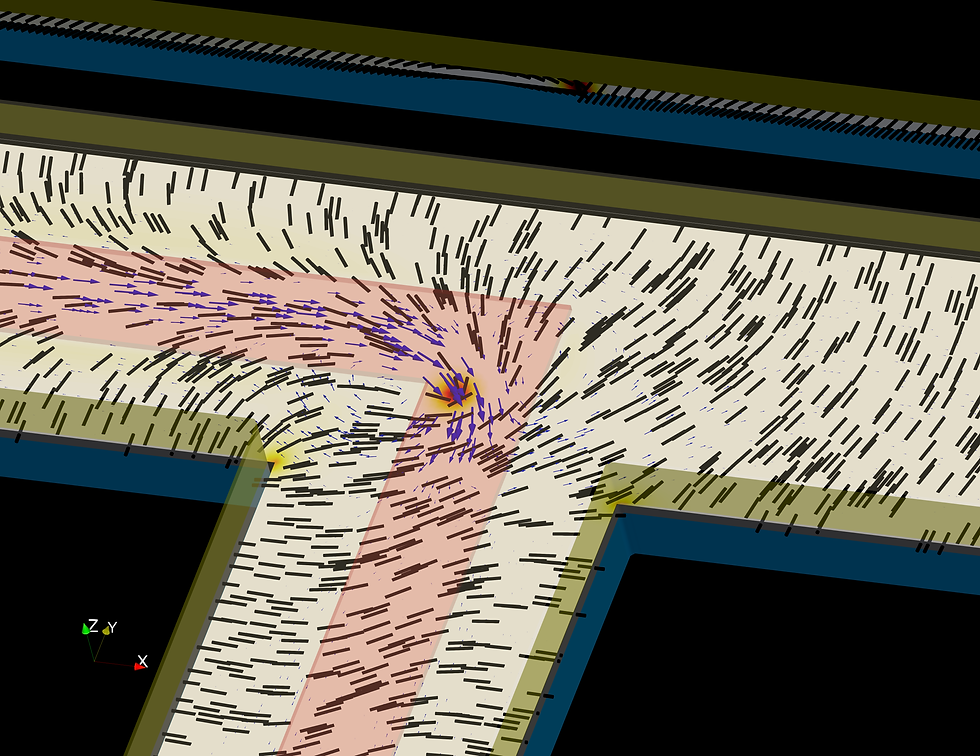
Our Research
Our society is currently facing unprecedented challenges in health, energy, and environment. And there is a strong demand in new materials which are renewable, multifunctional, light-weight and can interact with human more safely and more intelligently. Soft materials are a promising candidate for the above purpose. The overarching goal of the Computational Soft Matter Group is to harness soft materials, such as active matter, multiphase or porous media, liquid crystals, polymers, colloids, and mechanical metamaterials, to design next generation, autonomous materials and soft machines.
Specifically, our group will employ traditional and emerging computational methods, including machine learning, to propose novel soft materials with nontraditional functionalities, features and dynamics. Examples include active fluids with tailorable flow patterns, multiphase systems sensitive to specific stimuli, and origami materials with novel shape-changing behaviors in response to external fields. These new soft materials could find applications in soft robotics, wearable devices, space exploration, 4D printing, energy harvesting, smart buildings, sensing and diagnosis, and etc.
Our group strives to borrow the wisdom from biological systems and design synthetic materials and machines that are low-cost, green, biocompatible and intelligent. Our research is multidisciplinary, covering Physics, Biology, Chemistry, Materials Science, Chemical Engineering, and Mechanical Engineering.